Tools You Should Learn to Become an AI (Artificial Intelligence) and ML (Machine Learning) Master
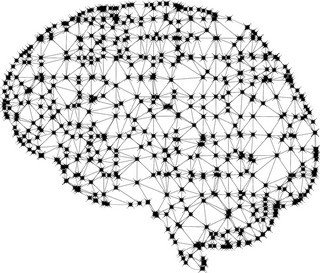
Some sixty years ago, artificial intelligence was just a concept that research scientists had in mind. But ever since the idea of super-computers-capable-of-thinking-like-humans has been floated, it has occupied a particular part in the public consciousness. Over recent years, we have seen tremendous growth and rapid evolution of artificial intelligence. Today, there is a vast amount of high-quality open-source libraries and software tools available to AI and ML experts. Every day, new ideas and concepts on AI are being discovered, and new applications of AI are being explored. We see how AI is slowly being used in business and everyday life. According to Ottawa IT services experts from Firewall Technical, AI technology will continue to be a significant force in many IT solutions in the next few years. Many tech experts agree that AI has a very bright future ahead, and some even predict the drastic changes AI can bring to the future generations. Considering all these great news, now is the best time to become an AI master. But for you to become an AI expert, you’ll need to learn some valuable tools in building AI algorithms.