The goal of any imaging methodology used in dermatology is to diagnose melanoma in the early stages because it depends on treatment effectiveness. Investigations show that early diagnosis is more than 90% curable and late is less than 50% [1]. The diagnosis and successful treatment are often supplemented with permanent monitoring of suspicious skin lesions.
The doctor’s diagnosis is reliable, but this procedure takes lots of time, effort. These routines can be automated. It could save lots of doctor’s time and could help to diagnose more accurately. Besides using computerized means, there is an excellent opportunity to store information with diagnostic information to use it for further investigations or create new methods of diagnosis.
Skin lesion imaging methods
We found that there are several various imaging methods of skin lesions [2]. The simplest skin visualization method is photography. This method gives only the top layer of the skin image. To get a deeper layer image, there is oil immersion used. It reduces reflections of the surface and brightens the image of the epidermis – the second skin layer.
Better results are reached when photos are made with a polarized light source. Then there are diminished reflections of light from the stratum corneum (top layer of skin). Then it is easier to estimate the lesion structures like dots, globules, nets that are the primary indicators of melanoma diagnosis.
The different illumination methods called epiliuminescence can be used to get the image from deeper skin layers. The light is directed straight into these layers and reflected through the lesion, giving more information about light absorbers’ consistency in these layers. This method of illumination improves the diagnostic accuracy of up to 10 percent [2].
Another interesting solution to getting more information from the skin is using multispectral photography [2]. There are used a narrow frequency band of light illumination. Those images give information about the consistency and concentration of absorbers and reflectors in the skin. The idea is that different skin pigment absorbs different light wave, determining the color of our skin. When those photos are made with a range of light waves, we can calculate the skin’s reflectance frequency characteristics. And compared to normal skin characteristics, there can be made diagnostic decisions about skin pigment consistency.
Another skin imaging method using a laser is called CLSM (confocal scanning laser microscopy). It uses a red or near-infrared low power laser beam to scan the skin surface. This beam can be focused into different deep to get an image of it. The deep is limited to 300µm because of absorbance [2]. The distance between two layers (axial resolution) can be about 2 – 5 µm. The main disadvantage of this method is the complicated acquisition of images from the reflected laser beam.
Ultrasound visualization is usually used to measure the depth of melanoma [1]. The other uses of ultrasound are limited by minimal tissue differences between healthy skin and lesion. If there is no melanoma practically, there are no differences. When the doctor diagnoses melanoma, he uses high-frequency ultrasound (over 30 MHz) to measure penetration depth to correct cut during surgery.
In optical coherence, tomography is used short near-infrared light pulses focused on the papillary dermis [2]. The reflected light is combined with reference light reflected from the mirror system to determine the depth of the papillary dermis. Measurement of the interference pattern allows the determination of the position within the tissue where the light was reflected. Using recent technologies with ultra sort light pulse, the maximum obtained resolution is 2 – 4 µm. Visualization depth is 1 – 1.5 mm.
The wide variety of methods shows that there is no best universal visualization. Some of them are used for different needs; others are costly. The choice of method depends on what features of skin lesions are wanted to visualize and the availability of resources.
Algorithms of skin image processing
Digital dermatoscopic images themselves do not provide formal and determining information. To get diagnostic information, digital image processing is used. Commonly used methods are based on geometrical feature extraction from an image with the lesion. The USA national health institute offers ABCD rule to classify dermatological images into benign, suspicious, and melanoma [3]. ABCD is the letters of first feature words: A (asymmetry), B (border), C (color), D (dermatoscopic structures). According to these four values there are total dermatoscopic value calculated by formula: TDV = A·1,3 + B·0,1 + C·0,5 + D·0,5 (1)
This score TDV contributes to the differentiation between benign and malignant lesions: 1,00 – 4,75 – benign skin lesion, 4,75 – 5,45 – suspicious, more than 5,45 – melanoma.
According to the “Romedix” organization, there is a presented comparison table of software solutions, using the ABCD rule.
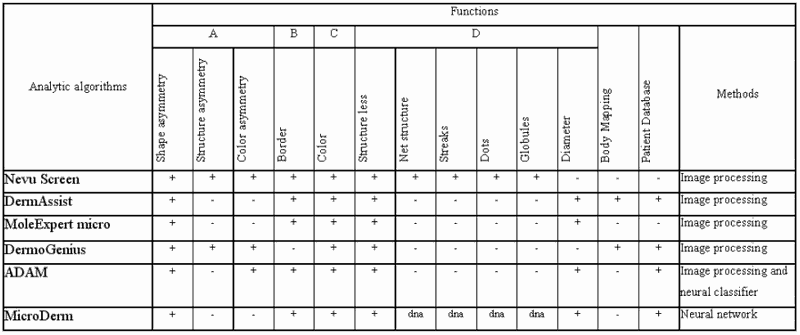
N – Not known
Most of those software solutions have some major disadvantages. First of all, they analyze geometrical and structural or color changes extracted from the plain surface picture, which does not contain enough useful information. The analysis is not connected with the physical nature of skin image. There are no associations with histological parameters of skin.
Another disadvantage is that diagnostic results do not always support diagnosis decisions. If there is the support of databases, then those pictures with diagnostic results are stored and used in decision support algorithms. Nowadays, there is a tendency to request past data and knowledge to better diagnose future results.
Digital dermatoscopic images firstly have to be parametrized for automatic classification. An in-depth study of skin nature has to be done before to parametrize it. It is beneficial to use a multilayer skin model [5].
The most common is the four-layer skin model: Stratum Cornea, Epidermis, Papillary dermis, and Reticular dermis.
Stratum Corneum is a top thin layer, which is a protective layer consisting of keratin-impregnated cells, and it varies considerably in thickness. Apart from scattering the light, it is optically neutral.
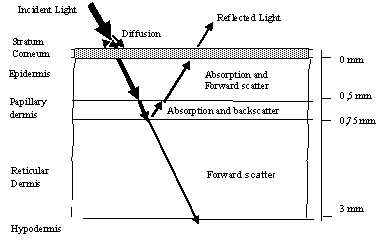
The epidermis is largely composed of connective tissue. It also contains the melanin-producing cells, the melanocytes, and their product, melanin. In this layer, there is a strong absorption of blue and ultraviolet light. Melanocytes absorb most of this light. It behaves like a blue and ultraviolet filter, which characteristics depend on the concentration of melanocytes. There is minimal scattering within the epidermal layer, with the small amount that occurs being forward directed. The result is that all light not absorbed by melanin can be considered to pass into the dermis. By this description, the epidermis can be characterized by wavelength-dependent coefficients: absorption coefficient of melanin mam(l) and melanin concentration cm [5].
The dermis consists of two sub-layers: papillary dermis and reticular dermis. The dermis consists of collagen fibers and, in contrast to the epidermis; it contains sensors, receptors, blood vessels, and nerve ends. The collagen fibers are thinner in the papillary dermis, and it behaves as a highly back-scattering layer. Any incident light is backscattered towards the surface. Scattering is greater in the red spectrum and going greater to infrared. Because infrared is not absorbed by melanin and blood, this part of the spectrum is best for assessing the papillary dermis’ thickness. The absorption coefficients can describe the papillary dermis for hemoglobin mah(l), the hemoglobin concentration ch—the scattering coefficient for collagen mspd, and the collagen layer’s thickness dpd.
Within the reticular dermis, the large size of collagen fiber bundles causes highly forward-directed scattering. Thus any light, which gets to this layer, is passed deeper into the skin and does not contribute to the spectrum emitted from the skin. The reticular dermis can be described by scattering coefficient msrd, and thickness of layer drd.
Model-based feature detection methods
The parameters describing skin pigmentation can be found by modeling light transport through a layered skin model. There can be used the Kubelka-Munk theory (Egan and Hilgeman, 1979). It calculates the remitted and transmitted light separately for each layer. And using the Beer-Lambert theory of light absorption, there can be calculated concentrations of pigments in each layer when reflectance coefficients are known [5].
This physical interpretation would let mathematically define the color changes because of melanin concentration changes in each layer.
The more significant part of melanoma diagnostic information is hidden in the dermis. In more than 90% of skin cancer cases, the dermal melanin causes melanoma [6].
The biggest problem is to define the melanin concentration in the reticular dermis because almost all light is back-scattered from the papillary dermis, and the rest of the light is forward scattered into deeper tissues almost without reflection. To solve this problem, there should help Beer-Lambert law combined with the Monte-Carlo simulation. Monte-Carlo simulation itself requires much of calculations but combining with Beer-Lambert theory. This time can be significantly reduced. When there are known characteristics of upper layers by using Monte–Carlo simulation, there can be calculated optical properties of the reticular dermis with adequate probability.
The main equations used in skin modeling calculations are [6]:
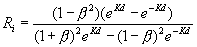
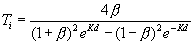
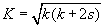

d – layer thickness, k ≈ μa – absorption coefficient, s ≈ μs- scattering coefficient of each layer, Ri and Ti – represents reflected and transmitted light intensities in i-th layer of the layered system.
Modeling enables to choose colors of lightning to obtain adequate spectroreflectoscopy images of skin lesions. Theoretically, it confirms the relations between histological features and features indicating colors in benign skin lesion images, suspicious lesion, or melanoma lesion. Skin photography imaging with adapted lightening and the model-based digital image processing, parameterization, and classification of skin lesion images provides objective images and estimates for early melanoma diagnosis. It contributes to the future of the dermatological decision support system.
Discussion
The survey shows the possible objective evaluation of skin lesion images based on established relations between histological features of lesion and color of its image. Mathematical modeling validates relationship. The future work is planned to construct a multicolor illumination adapter for widely distributed and cheap digital cameras, which might become affordable imaging and diagnosis tool for a wide range of dermatologists.
References
1. Valiukevičienė S. Melanomų diagnostika ir gydymas, Kaunas, KMU, 2002: 85p.
2. Ashfaq A. Marghoob, Lucinda D. Swindle, Claudia Z. M. Moricz, Fitzgerald A. Sanchez Negron, Bill Slue, Allan C. Halpern, Alfred W. Kopf, Instruments and new technologies for the in vivo diagnosis of melanoma New York, 2003.
3. D.D. Verma and A. Fahr, Confocal laser scanning microscopy study using lipophilic fluorescent probe DiI incorporated in liposomes for investigating the efficacy of a new device for substance deposition into deeper layers of the skin : Institut für Pharmazeutische Technologie und Biopharmazie, Philipps-Universität Marburg, 2001, https://www.dermaroller.de/images/NEEDLE.PDF.
4. W. Kopf, Skin oncology teaching center 2002 // https://www.dermoncology.com.
5. Leading Dermatological Imaging Systems Comparison Table, 2002 05 12// https://www.romedix.com/Comparison_table.htm.
6. Ela Claridge, Symon Cotton, Per Hall, Marc Moncrieff, From colour to tissue histology: Physics-based interpretation of images of pigmented skin lesions, School of Computer Science, The University of Birmingham, Birmingham, 2002, https://www.cs.bham.ac.uk/~exc/Research/Papers/.
7. Cotton S., Claridge E, Hall P., Detection the presence of dermal melanin through computer image interpretation, Abstract, Skin Research and Technology 1999.
That was an inspiring post,
I have been reading alot about melonomas and skin cancer and this has by far been the best resource,
Keep up the good work,
Thanks for writing, most people don’t bother.