Artificial Intelligence, or AI and machine learning (ML) has become increasingly important in many aspects of our lives. Machine learning is being used to analyze historical and fresh data to generate predictions and do tasks far more quickly.
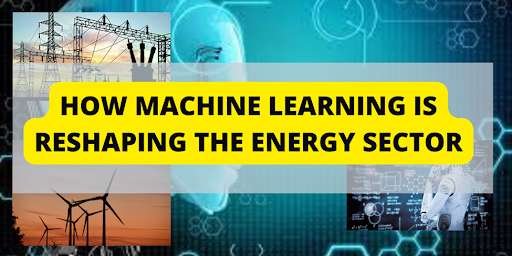
As a result, companies across the board are looking for ways to incorporate them into their core processes. This will help optimize and automate them, and the energy sector is no different!
ML has benefited renewable energy firms (wind, solar, hydro, and nuclear) throughout the years. ML is being used in the energy sector to improve energy efficiency by reducing consumption.
It is also used to enhance energy storage and power grid stability. Making forecasts about energy usage, and finding oil and gas with more precision, are also among its uses.
Uses Of Machine Learning In the Energy Field
Machine learning provides some capabilities to aid the energy sector. But how does ML specifically do that?
1. Energy Exploration and Drilling
AI is being employed in fossil fuel energy source exploration and drilling. In addition, it is also applied to weather forecasting and renewable energy source optimization.
For example, robots have been manufactured to survey the ocean’s surface. These robots use ML algorithms that allow them to learn from their mistakes while performing drilling.
This allows it to perform the same tasks as a scientist without danger. The robots help explore the ocean below, collect data, and analyze the information to help find new places to dig for oil and natural gas.
2. Eliminates the Release of Toxic Gasses During Energy Consumption
Google is well-known for its massive data centers that are located all over the world. These data centers produce a great deal of heat, which demands a lot of electricity to keep them cool.
ML model training and model monitoring are necessary to develop ML models. These models can be used in data centers for energy cooling, which helps save money on their power costs and reduce overall emissions.
3. Grid Management
One of the most exciting AI applications in the energy sector is grid management. Electricity is delivered to customers via a complex network, also known as the power grid.
Artificial intelligence and machine learning also play a key part in this field. These systems employ data analytics to forecast household energy consumption. The forecast is based on a certain period within a year, as well as data from past years.
This keeps power firms informed about the amount of energy needed in the next few days. They can control their grids without experiencing any outages as a result of this.
They can also increase energy production if demand is expected to be high. Alternatively, they can reduce production during certain times of the year when energy use is low to avoid waste.
4. Maintenance of Power Grids
Power grids, no matter how expertly managed, require repair from time to time. It is critical to keep the entire system running smoothly. The specific element of the system that requires maintenance can be easily anticipated using AI and machine learning.
Consumers can then be notified about grid maintenance when electricity companies are informed about upcoming maintenance work. Scheduled maintenance allows customers to be aware of impending power outages.
5. Forecasting the Weather for Energy Production
Renewable energy is undoubtedly the way of the future, but it also brings with it the risk of unpredictability. Renewable energy relies on natural resources such as sunshine, wind, and water.
All of these resources are intertwined with the weather, which humans have little control over. ML has aided in the resolution of this problem because it is a trustworthy instrument for weather forecasting.
It analyzes current weather and historical weather data using ML to deliver reliable forecasting. The forecast data is used by the power firms to manage the energy systems.
Companies produce renewable energy and store it if the weather forecast is favorable. If the weather forecast is bad, electricity firms adjust their load accordingly. They prepare for the problem and rely on fossil fuels to keep the electricity supply running.
6. For Prediction of Energy Prices
Consumers and businesses are increasingly producing their power sources. This is because personal power generation such as using solar or wind power becomes easier and cheaper.
People can generate, utilize, and store their energy through personal power generation. They may even be allowed to sell surplus electricity back to the local power utility, depending on where they live.
Machine learning can assist in determining the most advantageous time to create, store, or sell this energy. When prices are low, energy should be used or saved, and when prices are high, it should be sold back to the system.
Accurate predictions are made when we utilize machine learning models to look at past data, consumption trends, and weather forecasts. This aids individuals and businesses with energy generation systems in making strategic decisions about how to use their resources.
7. To Predict Energy Demand
Any utility company must be able to accurately estimate its customers’ energy needs. There is currently no acceptable option for bulk energy storage, which implies that energy must be delivered and utilized relatively immediately after it is generated.
Machine learning identifies complex patterns in a variety of influencing factors to explain demand fluctuations. These factors are:
- the day of the week
- time
- projected wind and solar radiation
- important sporting events
- past demand
- mean demand
- air temperature
- moisture and pressure
- wind direction
8. Optimization of Energy Consumption
People have long been aware of how much energy they use at home and work. We’ve only ever been able to get a general sense of energy use without doing a lot of manual computations, so we don’t know which appliances or devices use the most.
All of that has changed because too smart meters and the rise of the Internet of Things devices. Non-intrusive appliance load monitoring (NIALM), also known as disaggregation, is an ML-based technique that analyzes energy consumption at the device level.
Conclusion
AI and machine learning are transforming the renewable energy sector. These technologies have an impact on both power companies and consumers. Therefore, power companies can use ML to improve forecasts, grid management, maintenance schedule, and many other operations.
We are now seeing a lot of machine learning applications in the energy sector. It is fair to say that we should expect more of it in the future.