What is artificial intelligence if it isn’t doing what it’s supposed to? It’s like a kid on its first day of school: full of potential but as yet without the education to enact any of that potential. And how do we measure intelligence? With ongoing testing. Whether that’s the right way to go about it is for the school boards to decide, but when it comes to technology, it’s the best option we have. However, in order to test AI we need some metrics to test against, so what are the best markers for measuring artificial intelligence?
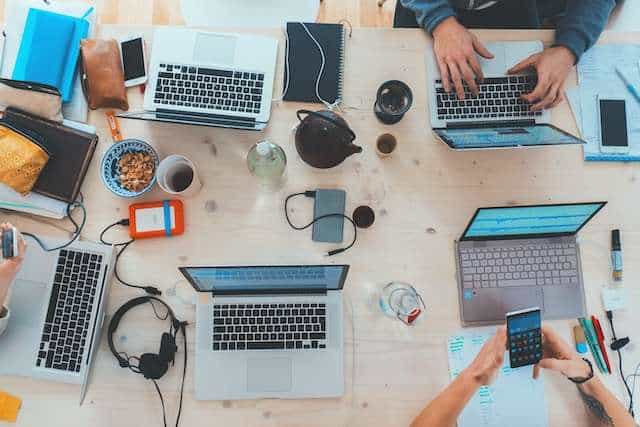
Take a look at our guide to find out.
Why do we need performance markers?
You would think that deciphering if a machine learning system was doing what it was designed to do would be obvious: did the machine do what the developer intended for it to do? But the answer is rarely so simple. Whether the answer is “yes” or “no”, developers need to look at how the system came to the conclusion it came to in order to determine if the system will continue to give the correct answer or if there is a reason it won’t. This will ensure a set of responsible AI principles.
And that’s where performance markers come in. Continuous testing and evaluation will allow machine learning system developers to understand where the system is going wrong and how to fix it for an optimal result every time.
Accuracy
As mentioned, the process of how a system came to a conclusion is important because there are actually four possible outcomes: true positive, true negative, false positive, and false negative. These factors measure the accuracy of the Artificial Intelligence system. If you were to get a true positive, it means the system took the correct steps and could perform the task you asked. A true negative means the correct steps were taken and something in those steps correctly blocked the result you asked for.
However, a false positive and negative means that something in the steps taken has incorrectly led to this result. It’s the equivalent of a student getting the right math answer but taking the wrong equation to get there. You’re asking the AI to show their work.
Precision and recall
Precision is all about how often a machine learning model gets the result right – and truly right. It’s a measure of the ratio of true positive outcomes against all positive outcomes. On the other hand, recall is a ratio of all positive predictions against actual positives, including false negatives. These aid in determining the accuracy and quality of your AI system.
User satisfaction
User satisfaction as a KPI is important to any product, even an AI system. However, they tend to involve their own set of KPIs, including active users, frequency of use, user behavior, session time, and more. Plus, they are affected by different factors, like whether the user got the result they wanted from the system, whether the system was easy to use, user interface, and other factors.
Business Impact
This is the most important if you are creating an AI system for a business. It’s a measure of what effect your AI system has on business outcomes, like reduced costs, higher sales, higher productivity and other core business objectives.